Microsoft SSIS: Transforming Enterprise Data Integration with SQL Server Integration Services
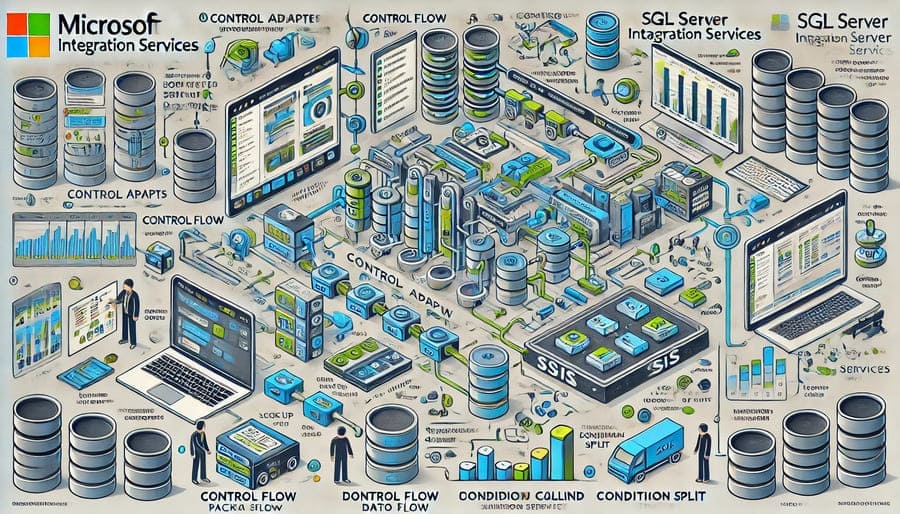
In today’s data-driven business landscape, organizations face a critical challenge: how to efficiently extract data from diverse sources, transform it to meet business requirements, and load it into target systems for analysis and decision-making. This Extract, Transform, Load (ETL) process forms the backbone of business intelligence and data warehousing initiatives, yet building reliable, scalable data integration pipelines remains complex.
Microsoft SQL Server Integration Services (SSIS) emerges as a powerful solution to this challenge, offering a comprehensive platform for enterprise data integration. As a core component of the Microsoft SQL Server data platform, SSIS provides organizations with robust tools to design, build, and manage data integration workflows that power critical business processes and analytics.
This article explores how Microsoft SSIS is transforming enterprise data integration, its key capabilities, implementation strategies, and real-world applications that can help your organization build more effective, maintainable ETL processes.
Before diving into SSIS’s capabilities, it’s important to understand the fundamental challenges in enterprise data integration:
Modern data environments present significant integration challenges:
- Source Diversity: Data spread across relational databases, flat files, APIs, cloud services, and legacy systems
- Volume Considerations: Processing requirements ranging from megabytes to terabytes
- Transformation Requirements: Complex business rules and data manipulations
- Performance Demands: Tight processing windows and SLAs
- Error Handling: Graceful management of exceptions and failures
These factors create substantial barriers to consistent, reliable data integration.
Maintaining and operating ETL processes presents ongoing challenges:
- Deployment Complexity: Managing development through production transitions
- Monitoring Requirements: Visibility into process execution and failures
- Restart/Recovery: Handling failures without complete reprocessing
- Scalability Concerns: Adapting to growing data volumes
- Maintenance Overhead: Updating processes as business requirements evolve
Organizations struggle to bridge this operational gap while keeping pace with changing business needs.
ETL development requires balancing various technical approaches:
- Coding vs. Configuration: Finding the right level of customization
- Reusability: Creating components that can be used across processes
- Standardization: Establishing consistent approaches to common problems
- Documentation: Maintaining clear understanding of process logic
- Knowledge Transfer: Enabling team members to support processes
Finding the right balance dramatically affects long-term success and sustainability.
Microsoft SQL Server Integration Services (SSIS) is an enterprise data integration platform that enables organizations to build high-performance ETL processes for data extraction, transformation, and loading. As a component of the SQL Server data platform, SSIS provides a visual design environment, robust runtime engine, and comprehensive management capabilities.
SSIS implements a comprehensive architecture designed for enterprise data integration:
+--------------------------------------------------------+
| |
| MICROSOFT SSIS |
| |
+----------------------+------------------+--------------+
| | | |
| Development Tools | Runtime Engine | Management |
| | | |
| +-----------------+ | +-------------+ | +----------+|
| | SSIS Designer | | | SSIS Service| | | SSISDB ||
| | - Control Flow | | | - Execution | | | Catalog ||
| | - Data Flow | | | - Logging | | | ||
| | - Parameters | | | - Variables | | +----------+|
| +-----------------+ | +-------------+ | |
| | | +----------+|
| +-----------------+ | +-------------+ | | Deployment||
| | Visual Studio | | | Packages | | | Utility ||
| | Integration | | | - XML Format| | | ||
| +-----------------+ | +-------------+ | +----------+|
| | | |
+----------------------+------------------+--------------+
- SSIS Designer: Visual design surface for building ETL processes
- Control Flow: Task sequencing and workflow orchestration
- Data Flow: High-performance data transformation pipeline
- Parameters/Variables: Configuration and state management
- Visual Studio Integration: Development within Microsoft’s professional IDE
- SSIS Service: Windows service managing package execution
- Package Runtime: Execution engine for SSIS packages
- Logging Infrastructure: Comprehensive activity logging
- Connection Managers: Abstraction for diverse data source connectivity
- SSISDB Catalog: Central repository for package storage and management
- Deployment Tools: Utilities for moving packages between environments
- Monitoring Capabilities: Tools for tracking execution and performance
- Security Framework: Integration with SQL Server security
SSIS provides several key capabilities that make it particularly valuable for enterprise data integration:
SSIS offers a comprehensive visual design experience:
- Drag-and-Drop Interface: Intuitive process construction
- Graphical Data Mapping: Visual field mapping and transformation
- Debug Capabilities: Testing and validation within the IDE
- Package Explorer: Navigating complex package structure
- Property Windows: Configuring component behavior
This visual approach accelerates development while improving clarity and maintainability:
CONTROL FLOW EXAMPLE:
+------------------+
| |
| Start |
| |
+------------------+
|
v
+------------------+
| |
| Execute SQL |
| (Truncate Table)|
| |
+------------------+
|
v
+------------------+
| |
| Data Flow Task |
| (Load Data) |
| |
+------------------+
|
v
+------------------+
| |
| Execute SQL |
| (Update Stats) |
| |
+------------------+
|
v
+------------------+
| |
| Send Mail Task |
| (Notification) |
| |
+------------------+
The heart of SSIS is its high-performance data transformation pipeline:
- Source Adapters: Connecting to diverse data sources
- Transformation Components: Manipulating data during processing
- Destination Adapters: Loading data into target systems
- Data Viewers: Monitoring data during development
- Buffer Architecture: Efficient in-memory processing
This data flow architecture enables sophisticated transformations with high performance:
DATA FLOW EXAMPLE:
+----------------+
| |
| OLE DB Source |
| (Customer Data)|
| |
+----------------+
|
v
+----------------+
| |
| Lookup |
| (Region Data) |
| |
+----------------+
|
v
+----------------+
| |
| Derived Column |
| (Calculate Age)|
| |
+----------------+
|
v
+----------------+ +----------------+
| | | |
| Conditional |---->| Bad Data |
| Split | | Destination |
| | | |
+----------------+ +----------------+
|
v
+----------------+
| |
| Aggregate |
| (Summary Data) |
| |
+----------------+
|
v
+----------------+
| |
| OLE DB |
| Destination |
| |
+----------------+
SSIS connects with virtually any enterprise data source:
- Relational Databases: SQL Server, Oracle, DB2, MySQL, PostgreSQL
- Files: Excel, CSV, XML, Flat Files, JSON
- Cloud Services: Azure, AWS, Salesforce, Dynamics 365
- Applications: SAP, Siebel, PeopleSoft, JD Edwards
- Big Data: Hadoop, MongoDB, Cassandra
- APIs: REST, SOAP, OData
This connectivity creates a universal integration fabric across the enterprise.
SSIS provides rich options for manipulating data:
- Lookup Transformation: Enhancing data with reference information
- Derived Column: Calculating new values with expressions
- Aggregate: Summarizing data with grouping operations
- Sort: Ordering data based on specified columns
- Merge/Merge Join: Combining data from multiple sources
- Conditional Split: Routing data based on conditions
- Script Component: Custom transformations with C# or VB.NET
- Slowly Changing Dimension: Managing dimensional history
- Fuzzy Lookup/Grouping: Handling imprecise matching
These transformations handle diverse processing requirements from simple mappings to complex business logic.
SSIS provides sophisticated control flow capabilities:
- Sequence Containers: Grouping related tasks
- Loop Containers: Iterative processing (For Each, For Loop)
- Task Library: Diverse processing operations
- File System Tasks (Copy, Move, Delete)
- Database Tasks (Execute SQL, Bulk Insert)
- Analysis Services Tasks
- Data Mining Tasks
- Scripting Tasks (PowerShell, VB/C#)
- Messaging Tasks (Send Mail)
- Precedence Constraints: Controlling execution flow
- Success/Failure/Completion constraints
- Expression-based conditions
- Multiple constraint combinations
This orchestration capability enables complex process workflows:
ORCHESTRATION EXAMPLE:
+---------------------------------------------------------------+
| |
| Sequence Container: Daily ETL Process |
| |
| +-------------------+ +------------------------+ |
| | | | | |
| | Initialize |------->| For Each File Loop | |
| | Environment | | | |
| | | | +--------------------+ | |
| +-------------------+ | | Process Single | | |
| | | File (Data Flow) | | |
| | +--------------------+ | |
| | | |
| +------------------------+ |
| | |
| v |
| +------------------------+ |
| | | |
| | Post-Processing | |
| | Tasks | |
| | | |
| +------------------------+ |
| |
+---------------------------------------------------------------+
SSIS provides flexible configuration capabilities:
- Package Parameters: Inputs that can be changed at execution time
- SSIS Environment Variables: Environment-specific settings
- Connection Managers: Abstracting connection details
- Expressions: Dynamic property values based on variables
- Configuration Files: External settings for packages
- Parent-Child Package Models: Hierarchical execution with parameter passing
This configurability enables flexible deployment across environments.
SSIS offers robust error management capabilities:
- Event Handlers: Responding to package events
- Error Outputs: Special paths for handling data errors
- Error Logging: Comprehensive error information capture
- Checkpoints: Restart capability for failed packages
- Transactions: Ensuring data consistency
These capabilities ensure reliable operation with appropriate error handling.
Successfully implementing SSIS requires thoughtful planning and execution:
Effective SSIS implementation follows structured development practices:
- Modular Package Design: Breaking processes into manageable units
- Template Packages: Standardized starting points
- Reusable Components: Common logic as shared objects
- Error Handling Standards: Consistent approach to failures
- Logging Strategy: Appropriate detail for troubleshooting
- Buffer Tuning: Configuring memory usage
- Parallelism Configuration: Appropriate task concurrency
- Batch Size Optimization: Balancing throughput and resource usage
- Lookup Optimization: Caching and memory management
- Connection Pooling: Managing database connections
- Development Lifecycle: Dev, Test, QA, Production progression
- Parameter Usage: Environment-specific settings
- Package Configuration: Managing environmental differences
- Source Control Integration: Version management for packages
- Deployment Automation: Streamlining environment transitions
SSIS supports multiple implementation models:
Suitable for simpler or standalone integration needs:
+-------------------+ +-------------------+ +-------------------+
| | | | | |
| Development | | Package | | Production |
| Environment |--->| Deployment |--->| Server |
| (Visual Studio) | | (.ispac files) | | (SSIS Catalog) |
| | | | | |
+-------------------+ +-------------------+ +-------------------+
Recommended for enterprise implementation:
+-------------------+ +-------------------+ +-------------------+
| | | | | |
| SSIS Project | | Project | | SSISDB Catalog |
| (Solution with |--->| Deployment |--->| (Environment- |
| multiple pkgs) | | (Project Model) | | specific config) |
| | | | | |
+-------------------+ +-------------------+ +-------------------+
Modern implementation with automated pipelines:
+-------------------+ +-------------------+ +-------------------+
| | | | | |
| Source Control | | CI/CD Pipeline | | Target |
| (Git/Azure DevOps)|--->| (Build & Deploy |--->| Environments |
| | | Automation) | | (Dev/Test/Prod) |
| | | | | |
+-------------------+ +-------------------+ +-------------------+
Maintaining a healthy SSIS environment requires operational discipline:
- Execution Logging: Tracking package execution
- Performance Monitoring: Identifying bottlenecks
- Failure Alerting: Notification of process failures
- Historical Analysis: Trend identification
- Execution Reporting: Visibility for stakeholders
- Package Auditing: Regular review of package inventory
- Performance Tuning: Ongoing optimization
- Version Management: Tracking package changes
- Backup Procedures: Protecting package assets
- Security Review: Access control verification
- Package Documentation: Clear purpose and process description
- Dependency Mapping: Understanding inter-package relationships
- Configuration Guide: Environment-specific settings
- Troubleshooting Guide: Common issues and resolutions
- Data Lineage: Source-to-target mapping documentation
Microsoft SSIS has been successfully applied across industries to solve diverse integration challenges:
A financial institution implemented SSIS for regulatory compliance reporting:
- Challenge: Consolidating data from 20+ systems for regulatory submissions
- Implementation:
- Built modular SSIS packages for each data domain
- Implemented comprehensive data validation and reconciliation
- Created multi-phase process with checkpoints
- Established detailed audit trail for compliance
- Developed automated error handling and notifications
- Results:
- 70% reduction in reporting preparation time
- Complete auditability for regulatory examination
- Enhanced data quality through automated validation
- Streamlined compliance with changing regulations
A healthcare provider used SSIS to streamline claims processing:
- Challenge: Integrating diverse claims data for processing and analysis
- Implementation:
- Developed standardized intake process for claims data
- Implemented complex validation rules
- Created provider and patient lookup enrichment
- Built exception handling for claims issues
- Integrated with claims processing system
- Results:
- 40% faster claims processing
- Reduced error rates through automated validation
- Enhanced analytics capabilities
- Improved provider and patient matching
A retail chain implemented SSIS for their merchandising analytics:
- Challenge: Building a comprehensive view of product performance across stores
- Implementation:
- Created incremental load processes for sales data
- Implemented slowly changing dimension handling
- Built complex product hierarchy transformations
- Developed store comparison analytics
- Created self-service reporting preparation
- Results:
- Near real-time visibility into product performance
- Enhanced inventory management through better data
- Improved markdown and promotion effectiveness
- More accurate forecasting and planning
Beyond basic ETL, SSIS offers several advanced capabilities:
SSIS provides powerful customization options:
- Script Task: Custom control flow logic with C# or VB.NET
- Script Component: Custom data flow transformations
- Custom Components: Developing specialized transformations
- Expression Language: Dynamic property configuration
- API Integration: Programmatic interaction with packages
This extensibility enables handling specialized requirements beyond standard capabilities.
SSIS works seamlessly with SQL Server’s Change Data Capture:
- CDC Control Task: Managing the CDC process
- CDC Source Component: Reading change data
- CDC Splitter: Routing based on change operation
- Incremental Load Patterns: Efficient processing of changes
- Historical Processing: Handling historical data loads
This integration enables efficient incremental data processing.
SSIS has evolved to work with cloud environments:
- Azure Feature Pack: Connectors for Azure services
- Integration Runtime: Running SSIS in Azure
- Azure Data Factory Integration: Orchestrating SSIS in the cloud
- Hybrid Scenarios: Connecting on-premises and cloud resources
- Azure-Aware Components: Working with cloud-native services
These capabilities bridge traditional and cloud-based integration.
SSIS can participate in big data scenarios:
- Hadoop Connectors: Reading and writing HDFS data
- Hive Integration: Working with Hive tables
- JSON/XML Processing: Handling semi-structured data
- Streaming Data: Processing high-volume data flows
- Scale-Out Execution: Distributing processing across servers
These capabilities extend SSIS to modern big data environments.
While SSIS remains widely used, Microsoft’s data integration strategy continues to evolve:
The relationship between on-premises and cloud integration:
- SSIS in Azure-SSIS IR: Lifting SSIS to the cloud
- Hybrid Execution: Combining cloud and on-premises processing
- ADF Orchestration: Managing SSIS within ADF pipelines
- Migration Paths: Transitioning from SSIS to ADF
- Coexistence Strategies: Using both platforms appropriately
Organizations are developing strategies that leverage both platforms.
Integration approaches continue to evolve:
- ELT vs. ETL: Shifting transformation to the target system
- Real-Time Integration: Moving beyond batch processing
- Data Virtualization: Logical rather than physical integration
- Metadata-Driven Approaches: Configurable rather than coded
- Self-Service Integration: Empowering business users
These patterns are influencing how organizations approach integration.
DevOps principles are being applied to data integration:
- CI/CD for ETL: Automated testing and deployment
- Infrastructure as Code: Managing integration environments
- Monitoring and Observability: Enhanced operational visibility
- Automated Testing: Validating integration processes
- Version Control Integration: Managing package lifecycle
This DataOps approach is bringing software engineering rigor to integration.
Organizations achieving the greatest success with SSIS follow these best practices:
Creating consistency across integration development:
- Develop standard templates for common patterns
- Create naming conventions for packages and components
- Implement error handling standards
- Establish logging requirements
- Document design patterns for reuse
This standardization dramatically improves maintainability.
Building efficiency through component design:
- Create reusable script components
- Develop shared connection managers
- Implement parameter-driven packages
- Build reusable transformation logic
- Create package templates for common scenarios
This approach accelerates development while improving consistency.
Ensuring quality through structured processes:
- Create test cases for packages
- Implement unit testing for complex logic
- Establish deployment verification
- Develop rollback procedures
- Document validation requirements
This testing discipline improves reliability and reduces issues.
Ensuring ongoing successful operation:
- Implement comprehensive monitoring
- Develop alerting for failures
- Create operational dashboards
- Establish maintenance procedures
- Document troubleshooting guides
This operational focus ensures sustainable long-term success.
In today’s data-intensive business environment, the ability to efficiently integrate data from diverse sources remains a critical capability. Microsoft SQL Server Integration Services (SSIS) provides a comprehensive platform for building, deploying, and managing ETL processes that power business intelligence, data warehousing, and operational data integration.
By combining visual development with powerful transformation capabilities, extensive connectivity, and robust operational features, SSIS enables organizations to implement enterprise-grade data integration solutions. From financial services to healthcare to retail, organizations across industries rely on SSIS for critical data processes.
While the data integration landscape continues to evolve—embracing cloud, real-time, and DataOps approaches—SSIS remains a valuable tool for organizations with SQL Server environments and established ETL practices. As part of a broader integration strategy that may include cloud-native tools like Azure Data Factory, SSIS continues to deliver the reliability, flexibility, and familiarity that many enterprise integration scenarios require.
For organizations seeking to establish or enhance their data integration capabilities, SSIS offers a mature platform with the depth and breadth to address diverse enterprise requirements while providing a bridge to emerging integration patterns and technologies.
#SSIS #SQLServerIntegrationServices #ETL #DataIntegration #MicrosoftSQL #DataWarehouse #DataTransformation #BusinessIntelligence #DataPipeline #DataEngineering #ETLProcess #SQLServer #DataFlow #MicrosoftBI #DataMigration #DatabaseIntegration #DataArchitecture #DataOps #EnterpriseDI #SSISPackage